Liangliang Cao is a principal engineer/director at Google. Previously, he worked as a scientist&engineer at Apple, Google, Yahoo!, IBM,
and an intern at Kodak, Microsoft Research, and NEC Labs. He co-founded a startup called Switi Inc., which was acquired in 2018.
Before the pandemic, he was an adjunct professor at UMass Amherst and earlier at Columbia University in New York.
He is an IEEE Fellow and also a recipient of the ACM SIGMM Rising Star Award.
Here is Liangliang's CV (updated April 2025).
Liangliang Cao
Researcher, Engineer, EntrepreneurPersonal email: llcao[at]cs.umass.edu
[LinkedIn], [X], [Google Scholar], [DBLP], [arXiv]
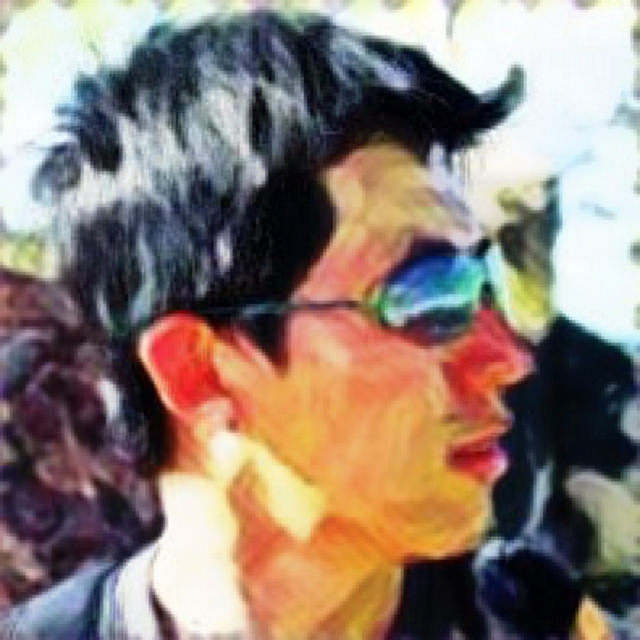
Bio
News
- New essay: Rethink Steve Jobs in 2025
- I am humbled and honored to be recognized as an IEEE Fellow.
Previous Projects
- Google DeepMind: Gemini Live, Astra, and Gemini Multimodality
- Apple Intelligence: modeling and engineering lead, 2023-2024
- Google Cloud Vision: tech lead to start the Cloud Multimodal Services, 2022
- Google Speech: team lead for Cloud speech modeling, 2018 - 2021
- Google-Verizon Contact Center AI: one of the largest contracts in the history of Cloud AI, 2019
- Switi Inc: Virtual Enterprise Representative Agent (Vera), 2016 - 2018
- ImageNet LSVRC Challenge: 1st place in 2010